Multi-modal large language models (MLLMs) are designed to process and understand data from various sources—text, images, charts, and more—enabling them to respond to complex, real-world queries with nuance and depth. But even the most advanced MLLMs still face challenges when it comes to truly aligning with human preferences, particularly when dealing with intricate images and detailed questions.
Enter OmniAlign-V and MM-AlignBench, two innovations that promise to redefine the way MLLMs are trained and evaluated. These tools aim to enhance the alignment of models with human values, making AI interactions more intuitive and contextually aware. Let’s dive into how these innovations are shaping the future of AI.
OmniAlign-V: A New Era in Multi-Modal Datasets
At the heart of OmniAlign-V is a massive, high-quality dataset designed to improve the performance of MLLMs in real-world, multi-modal environments. What makes this dataset so special? It's not just about the sheer size (although with 205K samples, it’s certainly substantial). It’s about the diversity and richness of the data.
Diverse Question Types for a Richer Dataset
OmniAlign-V doesn’t just focus on basic Q&A; it’s built to handle a wide array of question types, making it a powerful resource for training MLLMs that need to address complex scenarios:
- 39K Knowledge QAs: Factual questions that test the model’s ability to recall information.
- 37K Inferential QAs: Questions that require reasoning and deduction beyond simple facts.
- 10K Creative QAs: Tasks designed to assess creative thinking, where open-ended responses are key.
- 38K Instruction-Following QAs: Questions that test how well models can interpret and execute detailed instructions.
- 44K Infographic QAs: This unique category includes questions based on art, diagrams, charts, and other rich visual data.
But it’s not just the question types that set OmniAlign-V apart. The images associated with these questions are hand-picked for their semantic complexity. This ensures that the dataset doesn’t just include random visuals but images with detailed, meaningful content that enhances the context of each question-answer pair. This approach helps train models that are better at understanding and responding to nuanced multi-modal inputs.
Refinement Pipeline for Data Quality
OmniAlign-V is built on a solid foundation of data quality. A sophisticated refinement pipeline is used to ensure the highest level of accuracy and relevance:
- Filtering: Questions with significant discrepancies between model responses are filtered out.
- Merging: The best factual content from different models is combined, with a special focus on accurate OCR results.
- Human Expert Review: A final layer of human quality control guarantees the dataset's integrity.
This level of careful curation ensures that OmniAlign-V can be used to fine-tune models with confidence, knowing that the data is of the highest quality.
MM-AlignBench: The Benchmark for Multi-Modal Alignment
So, we’ve got an incredibly rich and diverse dataset, but how do we know if an MLLM is truly aligned with human values? Enter MM-AlignBench, an evaluation framework designed to test exactly that.
A New Kind of Evaluation Framework
MM-AlignBench is not just another benchmark; it’s a tool for measuring alignment between model outputs and human expectations in complex, multi-modal environments. The benchmark focuses on evaluating how well models handle complex charts, infographics, and detailed queries—areas where traditional models often fall short.
The evaluation process is straightforward but powerful:
- Win Rates: How often does the model get the right answer, aligning with human expectations?
- Rewards: How effectively does the model respond to multi-modal inputs, providing valuable, human-like responses?
What makes MM-AlignBench especially valuable is that it doesn’t just look at how accurate models are, but also at how human-like their responses are, ensuring that MLLMs are not just correct but also contextually aware.
Results: OmniAlign-V in Action
The combination of OmniAlign-V and MM-AlignBench leads to significant improvements in model alignment:
- Improved Alignment with Human Preferences: Fine-tuning MLLMs with OmniAlign-V shows a clear boost in the models' ability to align with human values. This means models can respond to complex, nuanced questions with a higher degree of accuracy and context.
- Addressing Human Alignment Degradation: Over time, many models suffer from a phenomenon called human alignment degradation, where their performance becomes less aligned with what humans expect. OmniAlign-V helps mitigate this by providing a rich, diverse dataset that reflects real-world scenarios more accurately.
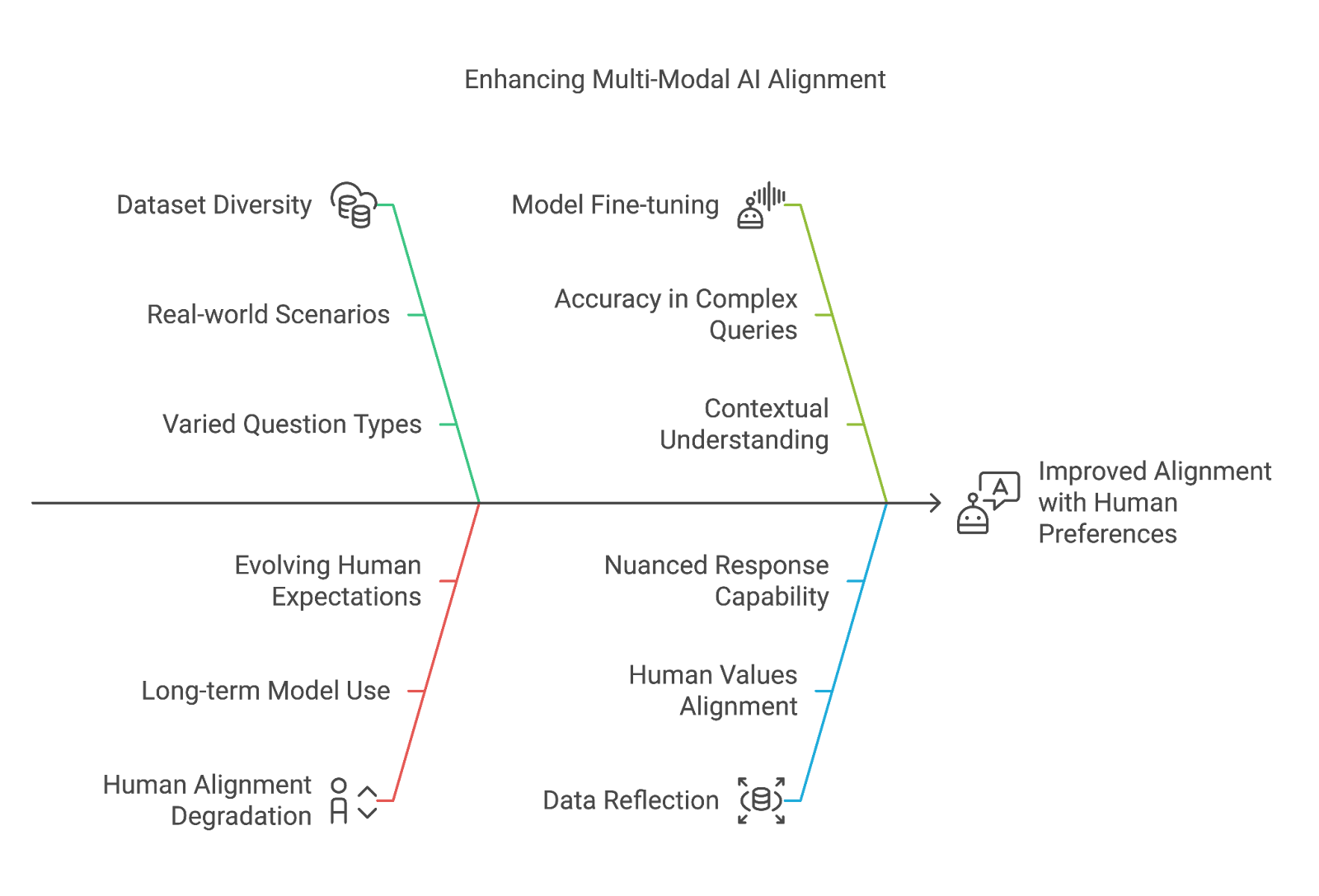
Conclusion: A Leap Toward Human-Centered AI
The introduction of OmniAlign-V and MM-AlignBench represents a major leap forward in the development of human-centered AI. By providing a high-quality, diverse dataset and a robust evaluation framework, these innovations are enabling MLLMs to become more aligned with human preferences—especially when dealing with complex, multi-modal inputs like images, charts, and detailed questions.
For AI to truly be useful in real-world applications, it needs to understand the complexities of human knowledge, reasoning, and creativity. OmniAlign-V and MM-AlignBench take us a step closer to that goal, helping to create models that not only perform well but also reflect the values and expectations of the humans they’re designed to assist.